In the rapid changing world of digitization, staying updated on technical advancement is a necessity both for outsmarting competition and achieving the desired business growth.
The term artificial intelligence or machine learning was generally considered to the highly technical terms and generally used by the tech-savvy person. The term was generally heard in the technical driver projects or in the science laboratories. However, in recent years, the finance sector has seen a steep rise in the use cases of machine learning applications. Technologies with artificial intelligence are integrating with financial services because of their exquisite performance of specific tasks specifically when raw unstructured data are involved. The artificial intelligence was primarily used by hedge funds but the last few years have seen the spread of the technology in banks, fintech, regulators and insurance firms.
Machine Learning works by extracting meaningful insights from raw sets of data and provides accurate results. This information is then used to solve complex and data-rich problems that are critical to the banking & finance sector. Further, machine learning algorithms are equipped to learn from data, processes, and techniques used to find different insights. Increased use of AI will allow accountants to:
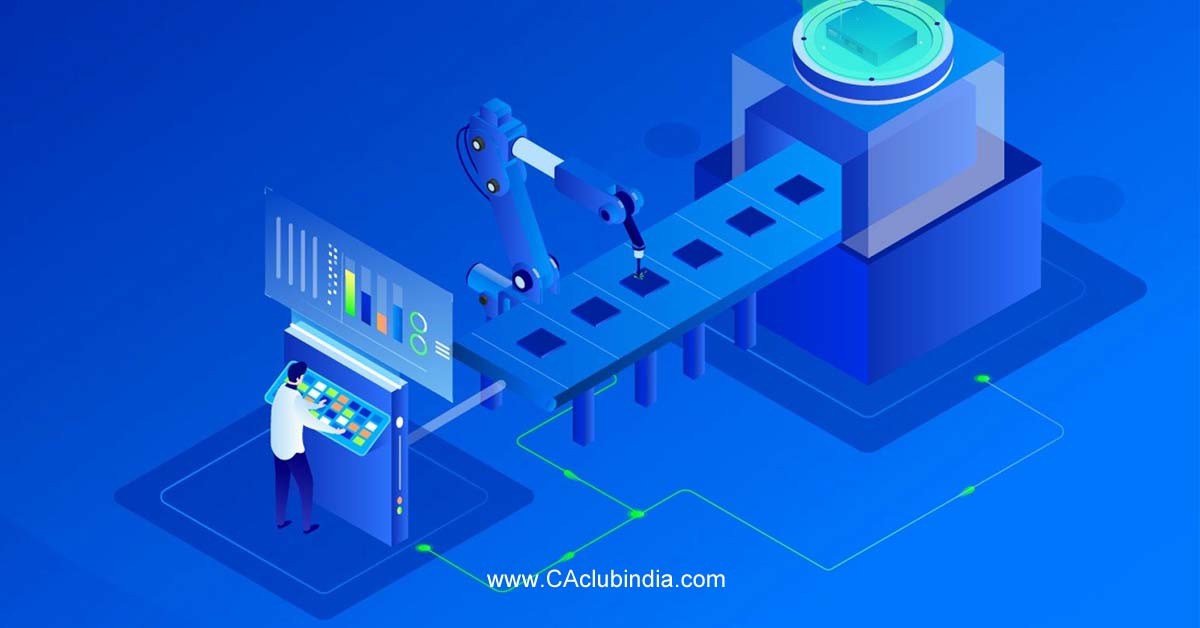
- Focus on giving better decision support rather than on data gathering and manual analysis.
- Gather and address associated risks with AI through productive governance and internal controls
- Look at both how we can support machine learning to facilitate our role as auditors and accountants
- Assist with the design of internal controls over their applications
- Guide other departments in their use of machine learning
Following are some of the USE CASES of Artificial intelligence in Finance:
1. Financial monitoring and red flags
Banks and insurance companies have thousand of gigabytes of consumer data which includes their personal and financial details. Some of the automated tasks like matching data records, looking for exceptions, detection of unusual activities and flagging red flag immediately, finding data and trends which people may generally miss and predicting the future patterns.
2. Investment Predictions
Artificial intelligent / machine learning enabled technologies provides market insight and allows the fund managers to identify specific market changes much earlier as compared to the traditional model. The AI reporting-based analysis helps to recommend auto savings and budgeting based on the spending pattern of the customer. They create customized advice based on new incoming data.
With renowned firms such as Bank of America, JPMorgan, and Morgan Stanley investing heavily in ML technologies to develop automated investment advisors, the disruption in the investment banking industry is quite evident.
3. Process automation on banking sector
Artificial intelligence helps to automate certain regular processes which can completely replace certain manual work performed. It enables automatic understanding of the new rules and regulations introduced, IBM Watson helps to understand the complex banking regulations, it helps to make loan decisions within few seconds based on the available data resulting in reduction in loan defaulters, answer financial research queries through best AI chatbot, paper work automation etc.
4. Risk management
Using the techniques of artificial intelligence and machine learning, financial institutions significantly lower the risk levels by analyzing massive volume of data sources. The insights help to provide subsequent decisions regarding the customer who are at the risk of defaulting their loans based on the spending patterns. The resultant would be a reduction in the customer service calls and fraud research cost. It can perform automated tasks like matching data records and looking for exceptions to analyze whether the applicant qualifies for loan or insurance.
5. Algorithmic trading / High Frequency Trading
Machine Learning in trading is another excellent example of an effective use case in the finance industry. Algorithmic Trading (AT) has, in fact, become a dominant force in global financial markets. ML-based solutions and models allow trading companies to make better trading decisions by closely monitoring the trade results and news in real-time to detect patterns that can enable stock prices to go up or down. Machine learning algorithms can also analyse hundreds of data sources simultaneously, giving the traders a distinct advantage over the market average.
6. Customer data Management
Banks, financial institutions and insurance companies has to handle huge customer data. The massive volume and structural diversity of financial data from mobile communications, social media activity to transactional details, and market data make it a big challenge even for financial specialists to process it manually. Integrating machine learning techniques to manage such large volumes of data can bring both process efficiency and the benefit of extracting real intelligence from data. AI and ML tools such as data analytics, data mining, and natural language processing, help to get valuable insights from data for better business profitability.
Roadmap for adopting Artificial Intelligence / Machine Learning
- Objective of implementing AI: Most of the organizations are not clear regarding the role of machine learning basically which activities are required to be automated. It requires breaking issues into smaller logical path, determine the performance KPI and success targets.
- Data to be used: Data source plays an important role as the volume of data. The data has to be segregated with respect to sensitivity. If the volume is not adequate, infrastructure to capture more data has to be established. Proper data protection protocols have to be implemented for this purpose.
- Training of the machine language: Train the model, range of factors that may affect the project to ensure important factors aren’t missed. The data and the exceptions which are needed to be analyzed is needed to be taken into consideration.
- Integration: Integration of the AI into the existing management system has to be taken into consideration. The existing management system should be able to adopt to the new algorithms introduced by the AI.
Conclusion
Although the finance sector has been little sceptical in use of machine learning / artificial intelligence, the last few years have proved us wrong and it has been widely used across various financial sectors. To sum up some of the pertinent reasons as to why financial sector should be using artificial intelligence.
- It helps to enhance revenue owing to better productivity
- Improves the customer experience
- Process automation leads to low operational cost
- Reinforce security and better compliance